Abstract
Objective: Computer-aided diagnosis (CAD) significantly enhances the accurate and early detection of breast cancer. The segmentation step, which is crucial in CAD methods, employs different algorithms for image segmentation as documented in the literature. Segmentation in detecting breast lesions using CAD may affect the features extracted from the images and, accordingly, the classification results. These segmentation methods have advantages and limitations when compared to each other. No study in the literature has yet explored feature matrices obtained by different segmentation methods using different performance criteria. This study aims to investigate the effects of different segmentation methods used in image processing on mammography images for breast cancer detection.
Materials and Methods: In the preprocessing step, images are enhanced using median filtering, Contrast Limited Adaptive Histogram Equalization (CLAHE), and unsharp masking. Texture features are extracted from the regions of interest (ROI) using texture analysis techniques, coupled with an elastic network technique for feature reduction.
Results: The performance of five different segmentation algorithms was compared using various performance measures such as accuracy, sensitivity, and specificity, alongside different classification methods. The k-means algorithm showed higher performance compared to other segmentation methods. It exhibited high efficacy, achieving an accuracy of 1.00 and 0.989 with Support Vector Machine (SVM) and Random Forest (RF) classifiers, respectively.
Conclusion: Segmentation methods used in image processing were found to have an impact on classification results. These computer-aided systems can be instrumental in patient classification.
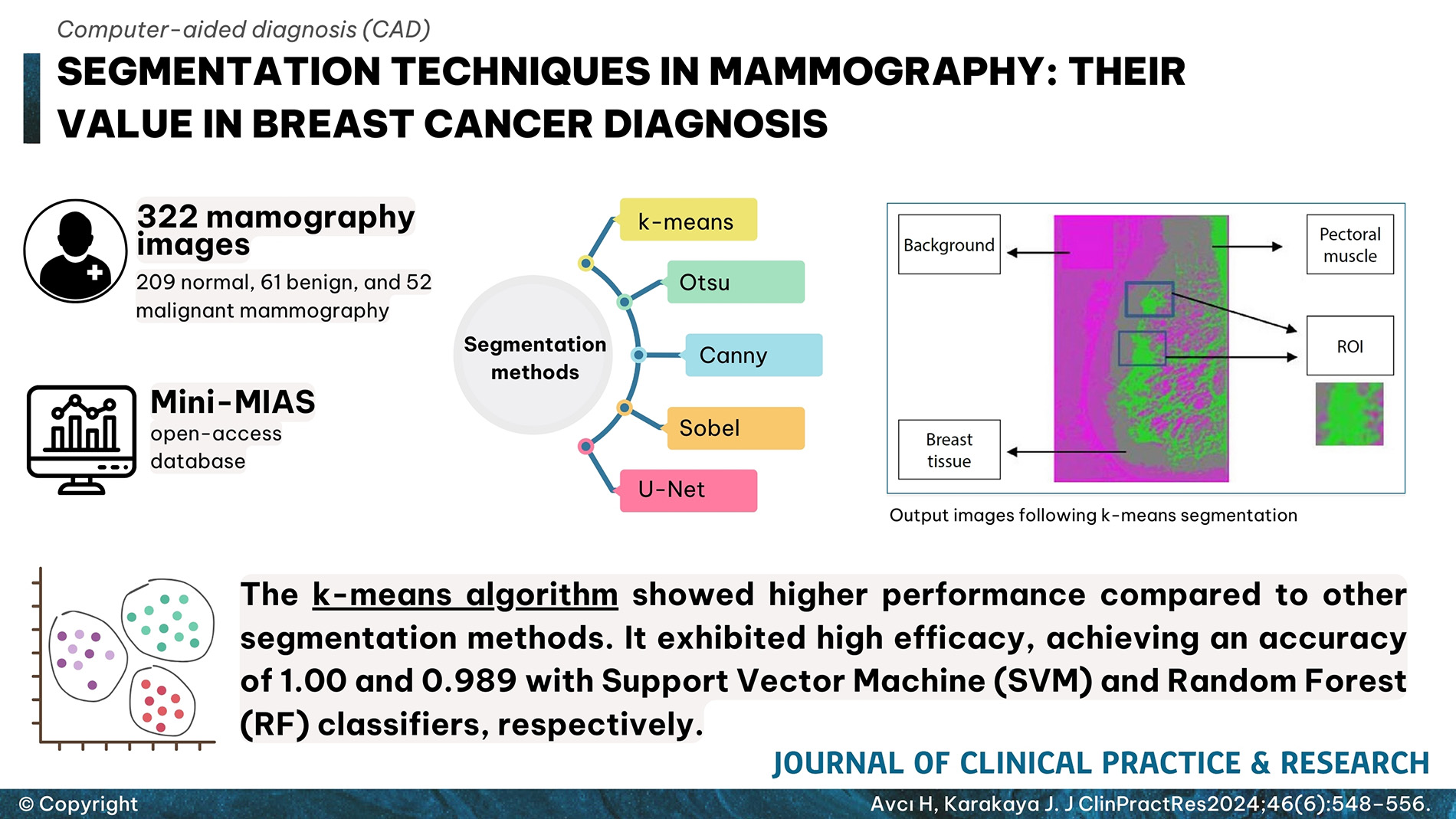